The new reality of data economy and productization: A conceptual paper
Abstract: The global rise of data volume from Zettabyte to Yottabyte raises concerns about effectively managing its use and storage. One concern is dark data, which needs to be tapped and used for critical decision-making and insights generation. This phenomenon is a reality in the data economy that requires a solution through productization. The term data economy refers to the expanding economic activity centered around data collection, analysis, and sale. With the digitization of society and the exponential growth of the internet and social media, individuals, organizations, and society are continuously generating and collecting massive amounts of data. This data can be invaluable for companies and institutions to gain insights into customer behavior, market trends, and the performance of their businesses. Despite the scholarly literature that provides some insights, there remains a need for further exploration and clarification of the intersection between the data economy and productization. This study addresses these unresolved issues by thoroughly analyzing academic and industrial literature. The study contributes to the existing body of knowledge by extending the TTF (Task-Technology Fit) theory to conceptualize the relationship between data economy and productization. The study also proposes a comprehensive framework for countries, organizations, and institutions and offers theoretical and managerial implications. It is important to note that this study has its limitations, and future research should address these limitations and further advance the understanding in this research domain.
Keywords: Data economy, productization, big data, conceptual paper, new reality
Authors:
Sunday Adewale Olaleye, corresponding author, Jamk University of Applied Sciences, School of Business, Finland, sunday.olaleye (at) jamk.fi
Akwasi Gyamerah Adusei, Industrial Engineering and Management, University of Oulu, Finland, akwasi.adusei (at) oulu.fi
1. Introduction
The data economy and productizing data have become increasingly important in today’s business and technological landscapes (Moilanen et al., 2023). Companies have realized the value of the data they collect and how it can be utilized through people, processes, and technology as the world becomes more digitized (Zhao et al., 2023). This digital transformation has generated and consumed massive amounts of data, creating new opportunities for businesses to monetize these resources (Brettel et al., 2023). Consequently, new business models and a stronger emphasis on data-driven decision-making have emerged. However, as the data economy evolves, it has brought forth new challenges and gaps that require further attention and exploration.
The data economy is a growing research field, particularly in regions such as Europe and America, while other continents like Africa still lag (Coyle & Li, 2021). Furthermore, literature on the data economy is scarce despite the efforts of companies and non-governmental organizations to publish non-academic articles. The data economy is a bridge between the past and the future, as evidenced by its value in 27 European Union countries and the United Kingdom, exceeding 440 billion euros in 2020. This sector has been steadily growing in Europe since 2016, with projections for 2025 ranging from 536.72 billion euros in the challenging scenario to 1,036.71 billion euros in the high-growth scenario (Statista, 2022a). However, despite the accelerated penetration of the data economy in certain regions, it has challenges. Security, privacy, regulations, government policies, and data volume control pose contenders to the data economy. Additionally, the challenges of data silos and dark data further compound the complexities faced by businesses.
The General Data Protection Regulation (GDPR), implemented in Europe in 2018, has played a crucial role in regulating privacy concerns within the data economy. As companies grapple with determining what data to control, customers who generate it also seek to have their voices heard. Despite the potential negative aspects of the data economy, it remains a new reality that has fundamentally transformed the dynamics of the data market. The data economy encompasses the overall impact of the data market on the economy, involving the generation, collection, storage, processing, distribution, analysis, elaboration, delivery, and exploitation of data enabled by digital technologies (Statista, 2022b). This definition highlights the significance of data as a driving force in the economy, emphasizing the importance of adhering to the FAIR (Findable, Accessible, Interoperable, and Re-usable) data principle. FAIR data is crucial for effective data sharing, serving as a bridge that connects countries across the globe in data generation.
The growth of worldwide data has been exponential, with data volumes increasing from Zettabytes to Yottabytes at lightning speed. This rapid data increase poses challenges regarding managing and utilizing it effectively. Another area for improvement is the existence of dark data, which remains untapped and underutilized for critical decision-making purposes. However, this dark data has the potential to be transformed into a commercially viable product through the process of productizing it. Additionally, the European Data Market (EDM) monitoring tool has been established to provide insights into data professionals, data market value, frequency of data suppliers, data user companies, their corresponding revenues, and the overall impact of the data economy on the European Union’s Gross Domestic Product (GDP). This project covers three years, from 2018 to 2020, and includes forecasts based on baseline, high-growth, and challenging scenarios till 2025. The data economy, if well-positioned, can achieve various goals, as Grover et al. (2018) mentioned in their study, including organizational performance improvement, business process enhancement, product and service innovation, boost in customer experience, and overall market enhancement. Ongoing academic debates surrounding the data economy explore various aspects, such as data ethics and the need for an acceptable data framework that protects consumers (Tett, 2021).
Different perspectives exist regarding the concept of data barter, as exemplified by the arguments of Tett (2021) and Omaar (2021). While Tett (2021) proposes transforming the discussion around data by adopting the concept of barter, Omaar (2021) refutes this idea by claiming that data is non-rivalrous and can be used simultaneously. Despite these debates, data has become a permanent fixture, holding immense potential for transformative societal and economic development. Valuing data remains a challenge, with differences in data protection laws across countries and changes in political office holders can impact the data gap and market participation (Coyle & Li, 2021). Data undergoes a metamorphosis, transitioning from raw data to information, knowledge, and understanding, eventually culminating in the creation of real products or services. This transformation highlights the value of data in driving constructive decision-making in business and other facets of life. The co-creation of value among data stakeholders is crucial for realizing the potential of the data economy and effectively utilizing big data (Coyle & Li, 2021). The study conducted by Haucap (2019) delves into competition policy in a data-driven economy, emphasizing the increasing use of data for designing products organizing, and monitoring production processes. Understanding the drivers and inhibitors of data is crucial, considering the multidimensional role data plays in data ecosystems.
Recent studies have approached the topic of the data economy from different angles. For instance, Olaleye et al. (2022) investigated the composition of the data economy using bibliometric methods and the Technology Conceptualization and Classification Matrix (TCCM) framework, while Olaleye and Adusei (2023) explored data culture as a scholarly discourse and examined the various dimensions of data culture and its significance in fostering innovation, facilitating informed decision-making, and enhancing competitiveness. Similarly, Donovan and Park (2022) explored the predatory inclusion of Kenyans through the data economy. However, these studies needed more detailed conceptualizations of the Data Economy. Other studies have examined the data economy holistically, highlighting issues such as privacy, security, trust, and the digital divide (McGraw et al., 2022).
Furthermore, van Erp and Swinnen (2022) focused on the legal aspects of the data economy. Lauf et al. (2022) combined data sovereignty and data economy, addressing tensions, and offering solutions. Despite the knowledge generated by scholarly literature, there still needs clarity regarding the intersection of the data economy and productization. This research aims to fill this knowledge gap by investigating the impact of the integrated data task technology fit model on the data economy and productization. Additionally, it explores the position of data productization in the data lifecycle and how it can be defined within the data product structure. The article is structured as follows: the second section delves into the economic significance of data, while the third section conceptualizes productization within the data economy. The fourth section summarizes the existing literature on data economy, productization, dark data, data management, and the integration of the task-technology fit theory. The fifth section outlines the methodology employed in this study, and the final section concludes with theoretical and managerial implications.
2. Commingling of data economy and productization
In today’s digital landscape, data generation is immense, with estimates reaching 2.5 quintillion bytes daily, as noted by Reboulet and Topping (2023). This staggering volume indicates the vast potential of data as an asset, far surpassing the magnitudes of national debts. Echoing this sentiment, Langdon and Sikora (2020) project an enormous market value for data, exemplified by the automotive sector, where car-generated data could be worth up to $750 billion by 2030.
The fusion of data economy and productization encompasses critical processes. Reboulet and Topping (2023) emphasize the foundational steps of recognizing data availability and mastering its extraction. They also highlight the necessity of robust data governance, defining it as a structured system for managing information-related processes. This perspective complements Langdon and Sikora’s (2020) view on data processing, which likens the current state of data productization to the early, unrefined stages of automobile manufacturing, suggesting a need for more sophisticated methods.
The concept of a ‘Data Factory’, introduced by Langdon and Sikora (2020), is central to this integration. It involves processes like data ingestion, harmonization, and quality scoring, thereby standardizing and rendering data products repeatable and comprehensible. This approach resonates with the views of Harkonen et al. (2019), who discuss the importance of structured, product-like data management in meeting diverse customer needs and treating data as a strategic asset. Data security and the productization of data desensitization technologies, as discussed by Wang et al. (2022), are critical in safeguarding data integrity and compliance in the data-centric business world. These considerations are vital for maintaining trust, especially under stringent regulations like the GDPR.
Olaleye et al. (2022) underscore the significant economic impact of data, predicting a global data economy worth $68 billion US dollars by 2025. The key to capitalizing on this potential lies in data productization, which involves creating structured product frameworks that clarify data offerings to stakeholders. Integrating Task-Technology Fit (TTF) theory, as suggested by Olaleye et al. (2022), is instrumental in aligning data capabilities with user requirements to optimize performance and data utilization. Synthesizing data economy and productization is an intricate yet advantageous venture. It demands a comprehensive approach that includes understanding data resources, establishing effective governance, forming structured data products, ensuring data security, and aligning data functions with user needs.
2.1 Economic relevance of data
It is imperative to swiftly address the challenges associated with data buying and selling regarding its exponential growth and financial worth. To tackle these issues, proper productization serves as a viable solution as it clarifies the offering and measurement of data (Glassberg, 2018). Various scholars have made efforts to establish frameworks for generating economic value from data in the academic realm. For instance, Opher et al. (2016) examine the “Rise of the data economy: Driving value through the Internet of Things data monetization,” highlighting that the data economy marketplace comprises data presenters, data insight providers, data platform owners, and data providers. Additionally, Falck and Koenen (2020) illustrate the data value chain, which involves the flow of data from collection and analysis to the data actors who generate value. Another noteworthy study by Zhao et al. (2019) offers a solution to three prominent issues in the big data market: data availability verification for customers, data providers’ privacy, and payment fairness. Zhao et al. (2019) propose a “new blockchain-based fair data trading protocol” as a potential remedy.
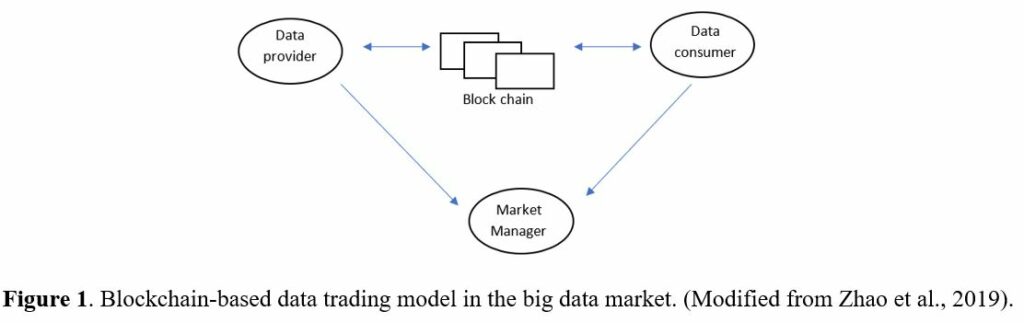
Figure 1 demonstrates the role of the market manager in facilitating communication between the data provider and the data consumer through registration. The data provider offers their published data topics on the blockchain after paying a deposit to the market manager. Subsequently, the data consumer requests specific data from the provider. The provider responds by sending encrypted data to the consumer. The customer then verifies the data by randomly challenging certain blocks, prompting the provider to respond accordingly. If the customer is satisfied, they can proceed with the purchase process. Once the purchase is made, the customer receives a Double-Authentication Preventing Signature (DAPS) signature from the data provider, allowing them to decrypt the data. Lastly, if the customer encounters any issues with the data, they can reclaim their deposit from the data manager.
2.2 Dark data
Data has been recognized as a critical asset in science and technology (Trajanov et al., 2018). The integrity of scientific research relies heavily on the data generated from experiments. However, much of this data remains unused and often hidden or lost (Heidorn, 2008; Trajanov et al., 2018). When companies know specific data, they often need help finding its relevance or value (Cafarella et al., 2016). One significant contributing factor to the abundance of unused data is the Internet of Things (IoT) trend, which has led to a massive increase in data due to the interconnectedness between IoT devices, sensors, and humans (Trajanov et al., 2018). Despite this exponential growth in data, most of it remains invisible and inaccessible to company managers (Gimpel, 2020). Shockingly, less than 1% of data is analyzed, with the remaining 99% being lost or in the dark (Trajanov et al., 2018).
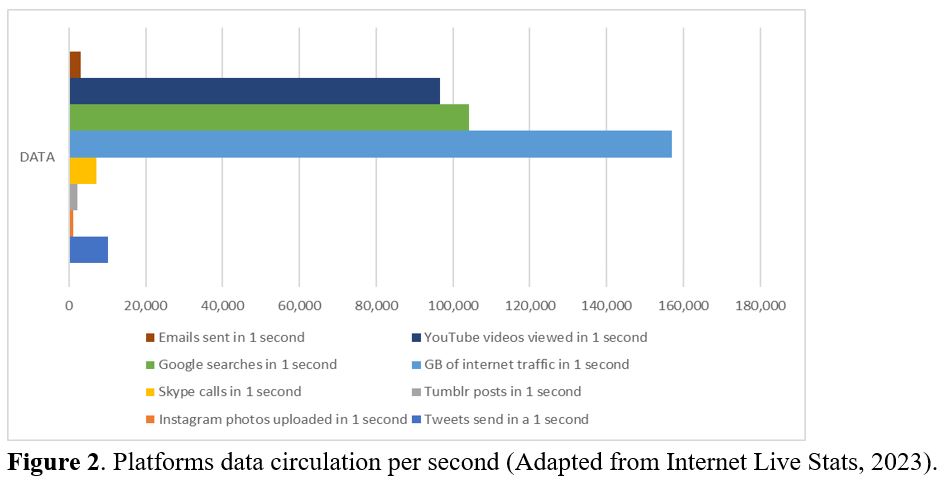
However, dark data holds immense potential. A study conducted by the University of Texas revealed that a 10% increase in data usability and remote access to data for a medium-sized Fortune 1000 company translates to a $2.01 billion increase in revenue and an additional $65.67 million in net income, highlighting the financial losses incurred by companies due to their dark data (Moumeni et al., 2021). Identifying and rectifying the forces that push data into obscurity is crucial to address these challenges, as suggested by Heidorn (2008). Moumeni et al. (2021) also propose a cost-effective approach to sorting, structuring, and visualizing data to uncover and utilize dark data. By gaining a better understanding of the data, companies can determine whether it is necessary to analyze it.
Different authors have proposed various definitions of dark data. However, this study aligns with Gartner’s definition, which describes dark data as “the information assets organizations collect, process, and store during regular business activities but generally fail to use for other purposes” (Gartner, 2023). Dark data is often unstructured, unlabeled, and untapped (Moumeni et al., 2021). The reasons for data going dark can vary, including the unavailability of time and computational resources and a lack of awareness of its contents (Munot et al., 2019). Given the significant importance of dark data, which constitutes a major portion of big data, it is imperative to take action to utilize it instead of leaving it in the dark. Leveraging as much available data as possible is crucial for reaping the benefits of machine learning, as valuable business insights may otherwise be lost or remain inaccessible (Trajanov et al., 2018).
2.3 Data management
In today’s increasingly data-driven world, the significance of data cannot be overstated, particularly when it comes to making strategic business decisions for growth. Technological advancements such as IoT, AI, and cloud computing have made data acquisition more accessible than ever, resulting in a staggering exponential increase in big data. This scenario can be illustrated by Figure 2, which depicts the new reality of data statistics according to Internet Live Stats (2023).
However, along with this exponential growth in data, there are also adverse effects to consider. Data silos, data explosion, and data security have emerged as challenges due to the overwhelming volume of data. If not managed properly, these challenges can lead to increased complexities for data enterprises (IBM, n.d.; Tolonen et al., 2014). One effective way to address these data bottlenecks is through efficient data management. The goal of data management is to cleanse and secure data to provide valuable insights. Gharaibeh et al. (2017) underline the fundamental importance of data in developing smart cities. Well-managed data enables consistency, interoperability, granularity, and reusability. Gharaibeh et al. (2017) view data management from three main perspectives: acquisition, processing, and discrimination.
Data management encompasses the practices of ingesting, processing, securing, and organizing an organization’s data, which is then utilized for strategic decision-making. As part of its data management strategy, IBM employs components such as data storage, data governance, data security, and data architecture. Data exploration, or e-science, represents the current and fourth paradigm in science and technology. This approach encompasses the previous three paradigms: experimental science, theoretical science, and computational science. The exponential growth of data from various sources, whether open or commercial, has facilitated the rapid advancement of machine learning algorithms and technologies (Trajanov et al., 2018).
2.4 Data productization
The classification of a product encompasses anything that can be sold to customers, as stated by Haines (2014). These products can be either tangible or intangible, according to Kahn (2012). Tangible products are physical, while intangible products encompass software-based offerings and services, as described by Hannila et al. (2019). Productization refers to creating a product, as Suominen et al. (2009) explain. Productization establishes consistent logic for any product offering, ensuring comprehension by all stakeholders (Harkonen et al., 2018). Harkonen et al. (2015) define productization as the systematic analysis of a need, definition, and combination of suitable elements, both tangible and intangible, into a standardized, repeatable, and understandable set of deliverables. A product structure serves as the foundation for the logic of productization, as highlighted by Adusei et al. (2021) and Lahtinen et al. (2019). It allows the modeling of a company’s products (Sudarsan et al., 2005). The product structure showcases the product itself, its data, its components, and the relationships between them (Saaksvuori & Immonen, 2008).
3. Data economy literature
The data economy has become a significant force in our modern society, transforming the role of the citizen-consumer and reshaping various aspects of our daily lives. This extant literature review highlights fundamental studies on different parts of the data economy. For instance, Lammi and Pantzar (2019) present a comprehensive overview of the digital turn’s impact on the role of the citizen-consumer. They emphasize the commercial dimension of consumer citizenship and examine how technological changes have influenced our lives. By focusing on new operational channels for citizen-consumers, the authors provide valuable insights into the evolving landscape of the data economy. While complementing this perspective, Engels (2019) zooms in on the importance of data governance in enabling the data economy. The author emphasizes the strategic significance of data as an asset and stresses the need for proper data governance practices. However, this study’s scope is limited to data governance, data management, and architecture, leaving out important aspects of data productization that could provide a more holistic understanding of the data economy. On the other hand, Börner et al. (2018) take a different angle and examine the skill discrepancies between research, education, and employment in the data economy. The authors highlight the growing demand for soft skills in the data-driven economy and the need to address these skill gaps in education and the labor market. This study underscores the importance of uniquely human skills, such as communication and negotiation, often overlooked in traditional education systems.
The personal data tsunami and its implications for the future of marketing were O’Neal’s priorities (2016). The study explores the transformative power of personal data and envisions a shift in marketing investments towards a people-data economy. By evaluating the effectiveness of investments in personal and social media data, O’Neal (2016) provides valuable insights into the real-time nature of this transformation. However, this study is limited in its focus on personal data within the marketing realm and may not capture the broader implications of the data economy. While each study reviewed offers valuable insights into specific aspects of the data economy, it is essential to recognize their limitations. The studies predominantly focus on dimensions such as citizen-consumers, data governance, skill dynamics, or personal data, missing out on the complexity of the broader data-driven paradigm. Therefore, a comprehensive and integrated understanding of the data economy is essential to capture its multifaceted nature fully. The literature reviewed in this study provides valuable insights into the various dimensions of the data economy. From the shifting role of citizen-consumers to the strategic importance of data governance and the need for soft skills in the data-driven economy, these studies contribute to our understanding of this transforming landscape.
3.1 Productization literature
In their study, Mansoori et al. (2023) focus on the concept of productization and how it can enable the successful implementation of Building Information Modeling (BIM) in the construction industry. The authors introduce the Part-Phase Matrix, a product structure specifically designed for the construction industry, by conducting conceptual research and a single case study. This matrix helps ensure consistency in the information exchanged through BIM. While the study provides valuable insights into productization and BIM implementation, it is essential to note that the findings are limited to a single case study, making it difficult to generalize the results to the broader construction industry. Therefore, further research is needed to validate and expand upon these findings.
On the other hand, Yrjönkoski and Systä (2019) delve into productization levels within the Software-as-a-Service (SaaS) industry. Through empirical research focused on a single case, the authors introduce a three-level phased productization model. This model highlights the different stages SaaS companies undergo as they evolve their offerings into whole products. While the study provides valuable insights into productization within the SaaS industry, it is essential to remember that the findings are limited to a single case study. This limitation underscores the need for broader industry assessments to validate and expand upon the proposed model.
Extant authors Lahtinen et al. (2019) contribute to the discussion on productization by examining commercial and technical productization within the context of product portfolio management. The authors introduce a comprehensive commercial and technical productization framework through qualitative research focused on a single case study. This framework provides valuable insights into managing product portfolios effectively. However, it is essential to note that the findings are limited to a single case company and specific product line divisions. Further research is necessary within broader industry contexts to ensure the comprehensive validation of this framework.
In their study, Artz et al. (2010) investigate the productization process in the software development industry. The authors propose a six-stage productization process using a combination of design science and case study research. While the study provides valuable insights into the transformation from customer-specific software to product software, it is crucial to recognize that the findings are based on a single case study. Consequently, further investigations are required to validate and refine the proposed productization process within a broader range of software development contexts. Also, Lahy et al. (2018) focus on productization within the logistics sector, particularly regarding developing a Product-Service System (PSS). Through an exploratory case study, the authors examine the driving and restraining forces influencing the adoption of a productization strategy in the 3PL industry. While the study provides valuable insights into the development of PSS and its decision-making process, limitations should be acknowledged. The study exclusively focuses on PSS and utilizes homogenous terms in search queries, which may limit the generalizability of the findings. Future research should consider a broader scope and diversified methodology to enhance the applicability of the conceptual framework developed in this study.
The literature reviewed herein highlights productization’s diverse and multifaceted nature across various industries. Each study contributes unique frameworks and insights into the concept. However, a common thread among these studies is the need for expanded research scope and diverse case validations. This approach is essential for fortifying the generalizability of the findings and ensuring their applicability within broader industry contexts. These consistent studies have paved the way for expanded future research.
Harkonen et al. (2017) propose a model for the product structure consisting of both a commercial and a technical product structure. The commercial product structure, organized hierarchically, includes levels such as the solution level, product family level, product configuration level, and sales items level. This structure is visible to customers. The technical product structure, on the other hand, is hierarchically arranged based on the tangibility or intangibility of the product. It includes product versions, main assemblies, sub-assemblies, and components for tangible products. The technical product structure comprises version items, main processes, sub-processes, cost drivers, and resources for intangible products.
3.2 Integrated theoretical framework of data task technology fit
The Task-Technology Fit (TTF) Theory is a theoretical framework that elucidates the connection between the characteristics of a technology and the tasks it is designed to accomplish. As per this theory, when there is a strong alignment between a task and its corresponding technology, both the technology’s performance and its users’ satisfaction will increase. In the data-driven economy, the TTF theory can be applied to ensure that data technologies and tools are well-suited to data-centric businesses’ specific tasks and objectives. This data-driven economy can be achieved by applying the principles of TTF theory to ensure that data technologies and tools are appropriately suited to the overall context in which they are implemented. For example, suppose a company intends to optimize its supply chain using machine learning. In that case, it must ensure that its machine learning algorithms are designed to handle the data inputs and business requirements specific to its supply chain operations. Failure to do so could result in inefficiencies within the supply chain.
Moreover, the TTF theory can help companies identify any weaknesses in their technological infrastructure that may impede their ability to leverage data effectively. By analyzing the fit between the technological needs of the business and the current technology in use, companies can determine areas where new technologies or tools are needed to support data-driven decision-making. TTF theory serves as a valuable tool for businesses aiming to maximize the value of their data assets within the data economy. By ensuring that the tasks performed align well with the technologies employed, companies can enhance their ability to generate insights and drive innovation.
The TTF theory, initially proposed by Goodhue and Thompson (1995), asserts that information technology is more likely to enhance individual performance and be utilized if its capabilities align with users’ tasks. Goodhue and Thompson (1995) assess various variables, including quality, locatability, authorization, compatibility, ease of use/training, production timeliness, systems reliability, and user relationship, as part of their task-technology fit evaluation. Each aspect is evaluated using a series of questions, ranging from strongly disagree to agree on a seven-point scale strongly. The interdependence between an individual (as a user of technology), the technology itself (comprising data, hardware, software tools, and associated services), and the task (the activity performed by individuals to achieve desired outputs) is referred to as task technology fit. The effectiveness of technology in facilitating user tasks is determined by the degree to which individual capabilities, task requirements, and technological features align harmoniously (Goodhue & Thompson, 1995).
Since its inception, TTF has been applied to various information systems, including electronic commerce systems, and combined with or used as an extension of other models related to information system outcomes, such as the technology acceptance model (TAM). The TTF measure, initially proposed by Goodhue and Thompson (1995), has been adapted and modified multiple times to suit the specific needs of various studies better. This study incorporates TTF into the data economy and productization context, considering factors such as data task characteristics, data platform characteristics, data technology task fit, data performance impacts, and data utilization.
3.2.1 Data task characteristics
The ubiquity of data in society necessitates understanding data task characteristics. Building upon the work of Spies et al. (2020), this study posits that data task characteristics encompass cognitive actions utilized in processing data at various task levels facilitated by appropriate technology. The principles of FAIR data, namely findability, accessibility, interoperability, and reusability, should exemplify these characteristics. Additionally, quality data, characterized by accuracy, completeness, reliability, relevance, and timeliness, is imperative. The study hypothesizes that Data Task Characteristics (H1) contribute positively to aligning data tasks with technology in an organizational context.
Data Platform Characteristics: An organization’s data platform comprises a comprehensive technology suite that supports end-to-end data requirements. It encompasses data acquisition, storage, preparation, delivery, regulation, and user and app security. Maximizing the value of data necessitates a robust data platform. Data Platform Characteristics denote the various channels through which organizations process their data, categorized into different types. Examples include enterprise data platforms catering to enterprise data assets, encompassing online transactional processing databases, data warehouses, and data lakes. Modern Data Platforms have evolved from electronic data processing and accommodate structured, semi-structured, and unstructured large-scale data processing.
This evolution is precious for organizations developing applications in artificial intelligence, machine learning, and natural language processing. Cloud Data Platforms offer dynamic storage and processing capabilities, enabling organizations to transfer or share data-related risks. They can manage unlimited data storage, massively parallel processing databases, and middleware for integration. Big Data Platforms fulfill the specialized needs of data analytics, integrating various big data tools and ensuring data availability, security, performance, and scalability. Additionally, Customer Data Platforms rely on customer-related data, consolidating sources such as social media, websites, customer relationship management, electronic commerce, and digital advertising data for analysis and insights. This study hypothesizes (H2) that utilizing a preference scale to order different data platforms based on their characteristics contributes to the alignment between data tasks and technology.
3.2.2 Data task technology fit
Technology plays a significant role in organizations, enhancing or supporting individual and group work and generating value. However, acquiring, deploying, and utilizing different technologies can be resource-intensive. When technology aligns with the intended data task characteristics it aims to support, it yields positive data performance impacts. These impacts encompass customer acquisition cost, revenue per customer, customer retention rate, customer satisfaction, revenue growth, profit margin, and customer data. This study hypothesizes (H3) that the alignment between data task characteristics and technology impacts data performance, thereby contributing to organizational revenue, customer retention, satisfaction, and growth.
3.2.3 Data utilization
Organizations use data for insights, decision-making, and revenue generation. This study hypothesizes (H4) that the alignment between data task characteristics and technology results in value creation for data utilization through data productization and monetization.
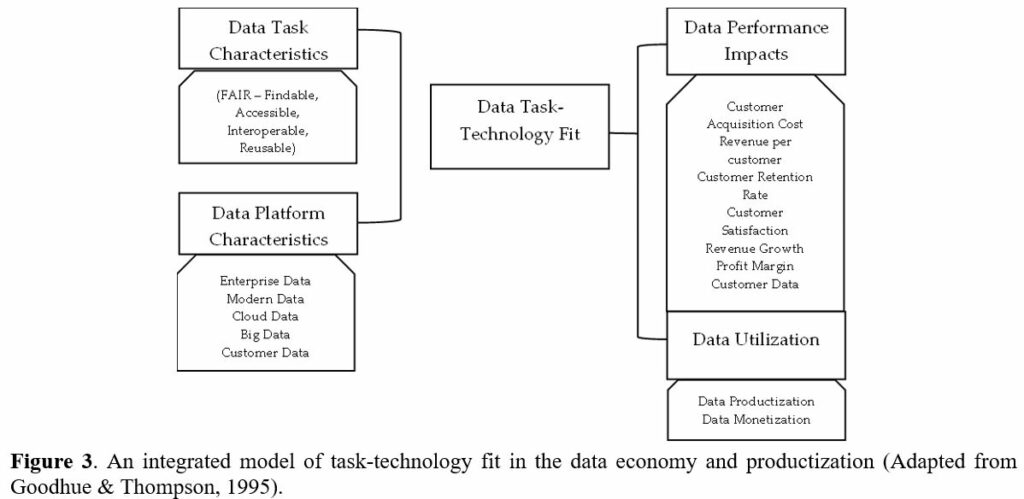
4. Methodology
This study adopts a qualitative research approach focused on conceptual analysis, synthesis of existing literature, and theoretical development. The study initially involves selecting and refining the research topic based on the authors’ prior knowledge and understanding of the data economy and productization domain. Further, the study utilizes detailed literature scrutiny to identify and analyze relevant scholarly articles, books, and other sources. The literature utilized in this study was obtained from Google Scholar and the institution’s subscribed databases, such as Web of Science and Scopus. Also, the study employs thematic analysis techniques to extract key themes, concepts, and theoretical insights from the literature and apply deductive reasoning to derive theoretical propositions and conceptual frameworks that contribute to advancing knowledge in data economy and productization. The scope of the database searches was limited to topics such as data economy, productization, data platforms, and task technology fit. Following this, the authors identified specific variables for the study. Finally, the authors developed a framework by integrating variables from scientific articles and other relevant materials to address the prevailing knowledge gaps within the field.
5. Discussion
5.1 Productization through data product structure model
Figure 4 presents a data product structure model adapted from the work of Harkonen et al. (2019). The model showcases the organization of data products within the commercial domain. The first level of the structure is the data solution level, which encompasses a wide range of data sectors. These sectors encompass various data categories, such as health, legal, and real estate data, represented by categories 1, 2, and 3, respectively. Each data category forms its own distinct data product family.
Moving further into the structure, we reach the data product configuration level. Here, each product category can offer different types of data tailored to meet customers’ specific needs.
Customers can select their preferences from a list of available data sales items. It is essential to highlight that the sales items level represents the final stage in the commercial data structure. This level consists of a list of data specifics that customers can choose from, some optional and others mandatory. Moreover, customers can choose from various optional and mandatory sales data. These selections enable customers to configure their data to align with their precise requirements. Sellable data can include raw, processed, or a combination of both. It is worth noting that customers only have visibility into the commercial product structure. On the other hand, the technical product structure pertains to the internal intricacies that lead to the formation of the commercial product structure. This structure includes data version items, processes and sub-processes, cost drivers, and the necessary resources. It is essential to bear in mind that the price of each sales item encompasses the cost of the technical version item in addition to the profit margin.
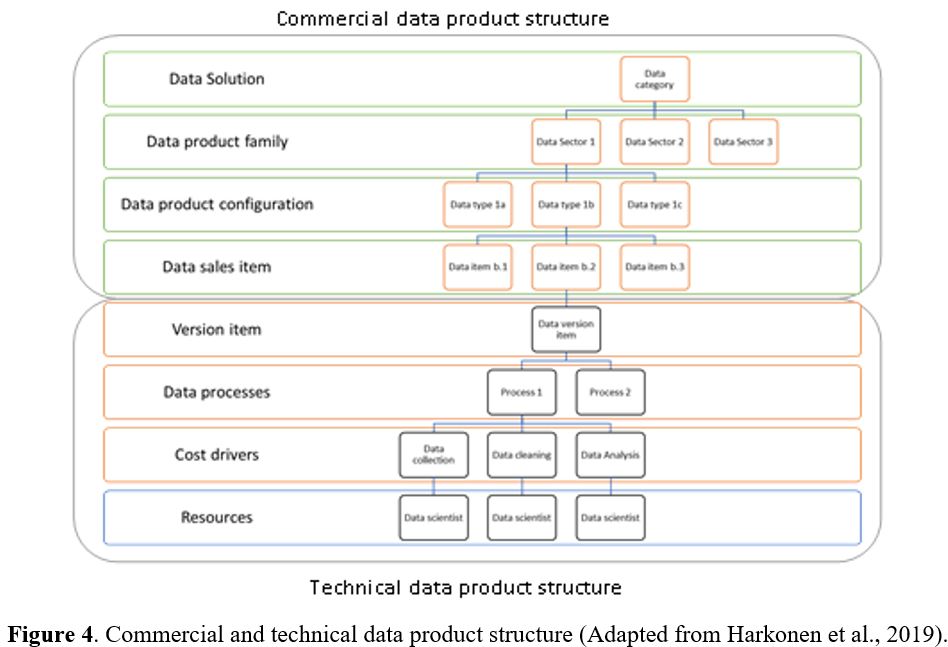
5.2 Framework for the new reality of data economy and productization
Figure 5 shows a proposed framework for the new reality of data economy and productization. It begins with data collection from diverse sources such as customer interactions, social media, and IoT devices. Once collected, data undergoes analysis using data mining and machine learning techniques to uncover patterns and insights. These insights are then utilized to create data categories that can be available for data productization, which involves creating new products or services, using data-driven applications, or leveraging data to design physical products. Businesses recognize the significance of data as valuable assets in the modern economy and seek to leverage them for value creation.
To effectively utilize data assets, businesses develop a data strategy that outlines goals, identifies metrics, and establishes data collection, analysis, and utilization processes. A robust data infrastructure is crucial to support data-driven initiatives, encompassing storage and processing technologies, and data visualization and analysis tools. As data usage becomes more prevalent, businesses must consider data ethics, encompassing privacy policies, data security, and transparency in data usage, ensuring responsible and ethical practices. By integrating these components, the data potential of companies can be unlocked to drive value creation and stay competitive in the data-driven economy.
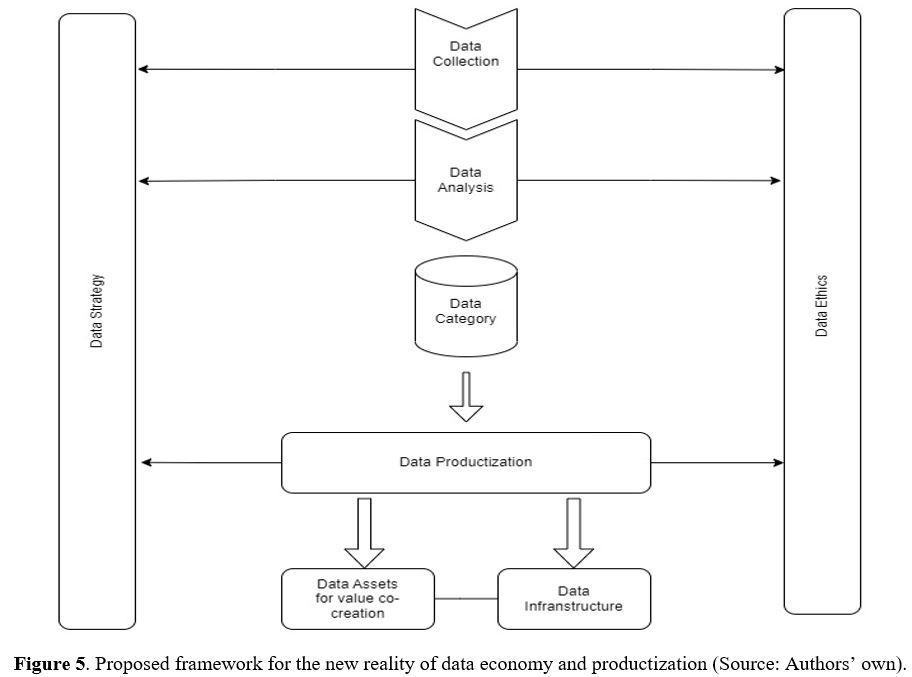
6. Conclusion
In this literature study, we aim to address a longstanding research question by demonstrating the potential positive impact of an integrated data task technology fit model on the global digital ecosystem. Numerous studies have already highlighted data’s economic significance and value in today’s digital economy. Therefore, it is crucial to carefully consider data, like any other marketable product, to maximize profitability and economic value. While productization has been extensively explored in terms of tangible and intangible products, the connection between the data economy and productization remains to be seen in current literature, despite the widely recognized economic value of data.
This study contributes to existing research by expanding the Task Technology Fit (TTF) theory to conceptualize the data economy and productization. The focus of this study is to propose a model for data productization through a structured approach. The significance of this study lies in its complementarity to ongoing research on productization (Harkonen et al., 2019) by providing an extended perspective from the data economy standpoint. The proposed model illustrates productization’s commercial and technical aspects in a hierarchical order, highlighting the interrelationships among different data structures.
Furthermore, our research demonstrates the applicability and integration of the data economy and productization within the Task Technology Fit framework. Specifically, we explore the characteristics of data tasks, data platforms, data technology task fit, and the impacts of data performance and utilization. The integration of the TTF theory aligns with a previous study by Wang et al. (2022), in which the authors integrate the TTF theory with IoT technology.
6.1 Theoretical and managerial implications
Spies et al. (2020) have previously noted the widespread application of TTF theory in various research fields, particularly in healthcare and mobile technology. This study offers a novel conceptualization of data economy and productization by integrating TTF theory into its framework to build upon existing research. This study holds great relevance and practicality for data product managers and the entire data monetization industry.
By utilizing the findings of this study, data managers can enhance their data management practices and maximize profitability. This study’s comprehensive data product structure provides a holistic view of data assets and offerings, allowing for more transparent communication between data stakeholders such as buyers, sellers, and platform managers. Moreover, the impact of data economy and data productization extends to managers in all industries. Firstly, businesses must prioritize investments in data management procedures as the value of data continues to grow. This investment entails ethical and secure data collection, storage, processing, and analysis throughout its lifecycle. Managers must deeply understand the data at their disposal, including its origin and potential applications in improving business operations.
Secondly, data-driven decision-making plays a crucial role in enhancing managerial judgment and strategic decision-making. Data analysis enables managers to identify patterns, forecast outcomes, and make informed choices. Consequently, managers must possess robust analytical skills and the ability to interpret data effectively.
Thirdly, the data economy opens opportunities for businesses to develop new products and services based on data, which can be achieved by creating data products. Managers must actively seek these opportunities and collaborate with their teams to design data-driven products that meet market demands and align with production capabilities.
Fourthly, to fully capitalize on the advantages the data economy offers, businesses must invest in data talent. This investment involves hiring data scientists, analysts, and engineers who can aid in data management and analysis. Managers should work closely with human resources to identify top-tier candidates and implement programs that foster ongoing education and professional growth.
Fifthly, as data becomes increasingly valuable, regulators are placing a greater emphasis on data privacy and security. Managers must ensure compliance with relevant regulations and safeguard their customers’ right to personal privacy. They must possess a deep knowledge of data regulations and establish robust data privacy and protection protocols. Lastly, the integration of data economy and productization presents opportunities for businesses to gain a competitive advantage. Also, to leverage data for decision-making and product development. However, managers must be cognizant of the challenges associated with data management and compliance in today’s data-driven economy. They must also invest in the right talent and technology to navigate this landscape effectively.
6.2 Future recommendations for countries, organizations, and institutions
More countries must appoint Chief Data Officers to lead their data strategies to thrive in the rapidly evolving data economy and productization landscape. Moreover, organizations should seriously consider the establishment of Chief Product Data Officers to monetize their data assets effectively. By embracing the four essential forces of data – people, process, technology, and data labor – data-driven initiatives can be strengthened, enhancing competitiveness in this new reality.
Drawing inspiration from the success achieved by Spain in appointing a Chief Data Officer, it is highly recommended that other countries emulate this approach (Capgemini, 2023). A Chief Data Officer is pivotal in managing and leveraging data as a strategic asset for economic growth and innovation. They guide data governance, data-driven decision-making, and data privacy and security. With the appointment of CDOs, countries, organizations, and institutions can ensure effective management and utilization of data resources, leading to valuable insights, informed policy-making, and economic competitiveness.
In addition to Chief Data Officers, it is strongly advised that both public and private organizations consider establishing Chief Product Data Officers (CPDOs). As data assumes the status of a prized commodity in the data economy, organizations must focus on effectively capitalizing on their data assets by transforming them into valuable products and services. CPDOs can drive the process of converting data into innovative offerings, ensuring that the data is adequately packaged, marketed, and delivered to cater to customer needs and generate revenue.
To further amplify data-driven strategies and initiatives, organizations must adopt a comprehensive approach that considers the four essential forces of data: people, process, technology, and data labor. In terms of people, organizations must invest in nurturing a data-driven culture by fostering data literacy, promoting data ethics, and enhancing data management skills among their employees. This proactive approach will empower individuals across the organization to effectively work with data and make informed decisions.
Regarding the process, organizations and institutions should implement robust data governance frameworks, establish stringent data quality standards, and develop efficient data management processes. Implementing such measures ensures data integrity, privacy, and compliance while facilitating effective data sharing and collaboration across departments and stakeholders.
Given the ongoing technological disruptions, organizations should embrace advanced tools and technologies for data processing, quality assurance, big data analytics, NoSQL Databases, knowledge discovery, stream analytics, in-memory data fabric, distributed storage, data virtualization, and data integration. These tools are often derived from artificial intelligence (AI) and machine learning (ML) and are essential for unlocking the full potential of data. Investing in data infrastructure and tools enables efficient data collection, storage, processing, and analysis.
Skills, competencies, and capabilities are crucial for effectively combining the realms of data economy and productization, and this is where the significance of data labor comes into play. Organizations must prioritize building diverse, multidisciplinary data teams because there is a need for more skilled data labor. These teams should comprise data scientists, analysts, engineers, and domain experts collaborating to extract valuable insights, develop data products, and drive innovation. By carefully considering and optimizing these four essential forces, organizations can lay a solid foundation for leveraging data as a strategic asset, fostering innovation, and generating economic value in the data economy.
6.3 Limitations and future studies
Our study is subject to certain limitations, particularly in the emerging data economy and productization areas. Acknowledging that our study adopts a conceptual approach, relying on analyzing secondary data collected from academic and grey literature. To further enhance the validity of our findings, the authors strongly urge empirical research in a case company where real case data products can be thoroughly examined, and the concept of productization can be applied. Furthermore, future researchers should empirically test the four hypotheses proposed in this study. Additionally, conducting quantitative tests on the integrated Theoretical Framework of Data Task Technology Fit and practically evaluating the suggested commercial and technical data product structure in various organizations across borders would yield valuable insights.
Acknowledgment
This work was supported by the Foundation for Economic Education (Liikesivistysrahasto), Finland [grant number: 24-13302], [grant number: 20-11445].
References
Adusei, A.G., Harkonen, J., & Mustonen, E. (2021). Productization and product structure: Extending the perspective to software business. International Journal of Business and Administrative Studies, 7(2), 89-106.
Artz, P., Weerd, I.V.D., Brinkkemper, S., & Fieggen, J. (2010). Productization: Transforming from developing customer-specific software to product software. International Conference of Software Business, 90-102.
Börner, K., Scrivner, O., Gallant, M., Ma, S., Liu, X., Chewning, K., … & Evans, J. A. (2018). Skill discrepancies between research, education, and jobs reveal the critical need to supply soft skills for the data economy. Proceedings of the National Academy of Sciences, 115(50), 12630-12637.
Brettel, M., Beule, T., Rey, M., & Huber, N. (2023). Monetization of machine-generated online data—Cross-industry opportunities and challenges. In The monetization of technical data: Innovations from industry and research, 87-102.
Cafarella, M., Ilyas, I. F., Kornacker, M., Kraska, T., & Ré, C. (2016). Dark data: Are we solving the right problems? 2016 IEEE 32nd International Conference on Data Engineering (ICDE), 1444–1445.
Capgemini (2023). CDE4PS conversations – Alberto Palomo, Chief data officer of Spain. https://www.capgemini.com/au-en/insights/research-library/cde4ps-conversations-alberto-palomo-chief-data-officer-of-spain/.
Coyle, D., & Li, W. (2021). The data economy: Market size and global trade. SSRN 3973028.
Donovan, K.P., & Park, E. (2022). Algorithmic intimacy: The data economy of predatory inclusion in Kenya. Social Anthropology, 30(2), 120-139.
Engels, B. (2019). Data governance as the enabler of the data economy. Intereconomics, 54(4), 216-222.
Falck, O., & Koenen, J. (2020). Resource “data”: Economic benefits of data provision. CESifo Forum, 21(3), 31-41.
Gartner (2023). What is dark data? https://www.ibm.com/topics/dark-data#:~:text=According%20to%20Gartner%2C%20dark%20data,business%20relationships%20and%20direct%20monetizing.&text=Most%20companies%20today%20store%20vast%20quantities%20of%20dark%20data.
Gharaibeh, A., Salahuddin, M.A., Hussini, S.J., Khreishah, A., Khalil, I., Guizani, M., & Al-Fuqaha, A., (2017). Smart cities: A survey on data management, security, and enabling technologies. IEEE Communications Surveys & Tutorials, 19(4), 2456-2501.
Gimpel, G. (2020). Bringing dark data into the light: Illuminating existing IoT data lost within your organization. Business Horizons, 63(4), 519-530.
Glassberg, S.E. (2018). How to build great data products. Harvard Business Review. https://hbr.org/2018/10/how-to-build-great-data-products.
Goodhue, D.L., & Thompson, R.L. (1995). Task-technology fit and individual performance. MIS Quarterly, 19(2), 213-236.
Grover, V., Chiang, R.H., Liang, T.P., & Zhang, D. (2018). Creating strategic business value from big data analytics: A research framework. Journal of Management Information Systems, 35(2), 388-423.
Haines, S. (2014). Product manager’s desk reference. McGraw-Hill Education.
Hannila, H., Tolonen, A., Harkonen, J., & Haapasalo, H. (2019). Product and supply chain related data, processes, and information systems for product portfolio management. International Journal of Product Lifecycle Management, 12(1), 1–19.
Harkonen, J., Haapasalo, H., & Hanninen, K. (2015). Productization: A review and research agenda. International Journal of Production Economics, 164, 65–82.
Harkonen, J., Mustonen, E., & Hannila, H. (2019). Productization and product structure as the backbone for product data and fact-based analysis of company products. IEEE International Conference on Industrial Engineering and Engineering Management (IEEM), Macao, China, 474–478.
Harkonen, J., Tolonen, A., & Haapasalo, H. (2017). Service productization: Systematizing and defining an offering. Journal of Service Management, 28(5), 936-971.
Harkonen, J., Tolonen, A., & Haapasalo, H. (2018). Modeling of manufacturing services and processes for effective productization. 20th International Working Seminar on Production Economics, Innsbruck, Austria, 19-23.
Haucap, J. (2019). Competition and competition policy in a data-driven economy. Intereconomics, 54(4), 201-208.
Heidorn, P.B. (2008). Shedding light on the dark data in the long tail of science. Library Trends, 57(2), 280–299.
IBM (n.d.). What is data management? https://www.ibm.com/topics/data-management.
Internet Live Stats (2023). 1 Second – Internet Live Stats. https://www.internetlivestats.com/.
Kahn, K.B. (2012). The PDMA handbook of new product development. John Wiley & Sons.
Lahtinen, N., Mustonen, E., & Harkonen, J. (2019). Commercial and technical productization for fact-based product portfolio management over lifecycle. IEEE Transactions on Engineering Management, 68(6), 1826-1838.
Lahy, A., Li, A.Q., Found, P., Syntetos, A., Wilson, M., & Ayiomamitou, N., (2018). Developing a product–service system through a productization strategy: A case from the 3PL industry. International Journal of Production Research, 56(6), 2233-2249.
Lammi, M., & Pantzar, M. (2019). The data economy: How technological change has altered the role of the citizen-consumer. Technology in Society, 59, 101157.
Langdon, C. S., & Sikora, R. (2020). Creating a data factory for data products. Workshop on E-Business, 43-55.
Lauf, F., Scheider, S., Bartsch, J., Herrmann, P., Radic, M., Rebbert, M. & Meister, S. (2022). Linking data sovereignty and data economy: Arising areas of tension. Wirtschaftsinformatik 2022 Proceedings. https://aisel.aisnet.org/wi2022/it_for_development/it_for_development/19.
Mansoori, S., Harkonen, J., & Haapasalo, H., (2023). Productization and product structure enabling BIM implementation in construction. Engineering, Construction and Architectural Management, 30(5), 2155-2184.
McGraw, D., Brillman, D., Ossowski, Y., & Padmanabhan, P. (2022). Consumer loyalty in the new health data economy: Fair data strategies, innovation, trust & trends: Panel session. Telehealth and Medicine Today. https://doi.org/10.30953/tmt.v7.347.
Moilanen, J., Luhti, T., & Niilahti, J. (2023). Deliver value in the data economy. BoD-Books on Demand.
Moumeni, L., Slimani, I., Farissi, I.E., Saber, M., & Belkasmi, M.G. (2021). Dark data as a new challenge to improve business performances: Review and perspectives. 2021 International Conference on Digital Age & Technological Advances for Sustainable Development (ICDATA), 216–220. https://doi.org/10.1109/ICDATA52997.2021.00049.
Munot, K., Mehta, N., Mishra, S., & Khanna, B. (2019). Importance of dark data and its applications. 2019 IEEE International Conference on System, Computation, Automation and Networking (ICSCAN), 1–6. https://doi.org/10.1109/ICSCAN.2019.8878789.
O’Neal, S. (2016). The Personal-data tsunami and the future of marketing: A moments-based marketing approach for the new people-data economy. Journal of Advertising Research, 56(2), 136-141.
Olaleye, S.A. & Adusei, A.G. (2023). Exploring bibliometric perspectives on data culture in scholarly discourse. The International Conference on Electronic Business, October 17-23, Chiayi, Taiwan.
Olaleye, S.A., Mogaji, E., Agbo, F.J., Ukpabi, D., & Adusei, A.G. (2022). The composition of data economy: A bibliometric approach and TCCM framework of conceptual, intellectual, and social structure. Information Discovery and Delivery, 51(2), 223-240.
Omaar, H. (2021). No, the data economy is not a barter economy. https://datainnovation.org/2021/09/no-the-data-economy-is-not-a-barter-economy/.
Opher, A., Chou, A., Onda, A., & Sounderrajan, K. (2016). The rise of the data economy: Driving value through internet of things data monetization. IBM Corporation.
Otto, B., & Österle, H. (2015). Corporate data quality: Prerequisite for successful business models. https://www.cdq.com/events-insights/publications/corporate-data-quality-en.
Reboulet, R., & Topping, C. (2023). Setting the stage for data productization. The Jabian Journal, Spring 2023, 25-29.
Saaksvuori, A., & Immonen, A. (2008). Product lifecycle management. Springer Science & Business Media.
Spies, R., Grobbelaar, S., & Botha, A. (2020). A scoping review of the application of the task-technology fit theory. Conference on e-Business, e-Services, and e-Society, 397-408.
Statista (2022a). Value of the data economy in the European Union (EU) and United Kingdom from 2016 to 2020 and in 2025*(in billion euros). https://www.statista.com/statistics/1134993/value-of-data-economy-eu-uk/.
Statista (2022b). Big data – Statistics & facts. https://www.statista.com/topics/1464/big-data/#topicOverview.
Sudarsan, R., Fenves, S.J., Sriram, R.D., & Wang, F. (2005). A product information modeling framework for product lifecycle management. Computer-aided Design, 37(13), 1399-1411.
Suominen, A., Kantola, J., & Tuominen, A. (2009). Reviewing and defining productization. 20th Annual Conference of the International Society for Professional Innovation Management (ISPIM 2009), Vienna, Austria.
Tett, G. (2021). The data economy is a barter economy. Harvard Business Review, https://hbr.org/2021/07/the-data-economy-is-a-barter-economy.
Tolonen, A., Harkonen, J., & Haapasalo, H. (2014). Product portfolio management – Governance for commercial and technical portfolios over life cycle. Technology and Investment, 5(4), 173-183.
Trajanov, D., Zdraveski, V., Stojanov, R., & Kocarev, L. (2018). Dark data in Internet of Things (IoT): Challenges and opportunities. 7th Small Systems Simulation Symposium, Nis, Serbia.
van Erp, S., & Swinnen, K. (2022). The legal status of co-generated data: With particular focus on the ALI-ELI Principles for a Data Economy and the rules on accession, commingling and specification. Technology and Regulation, 2022, 61-70.
Wang, H., Luo, X., & Yu, X. (2022). Exploring the role of IoT in project management based on Task-technology Fit model. Procedia Computer Science, 199, 1052-1059.
Yrjönkoski, T., & Systä, K. (2019). Productization levels towards whole product in SaaS business. Proceedings of the 2nd ACM SIGSOFT International Workshop on Software-Intensive Business: Start-Ups, Platforms, and Ecosystems, 42-47.
Zhao, W., Al Jneibi, L.H., Al Mashghouni, S.M., & Almheiri, O.O. (2023). Data analytical thinking: The new booster to petroleum industry and foundation of data-driven organization. SPE Gas & Oil Technology Showcase and Conference.
Zhao, Y., Yu, Y., Li, Y., Han, G., & Du, X. (2019). Machine learning-based privacy-preserving fair data trading in big data market. Information Sciences, 478, 449-460.